How Federated Learning is helping in Revolutionizing User Privacy
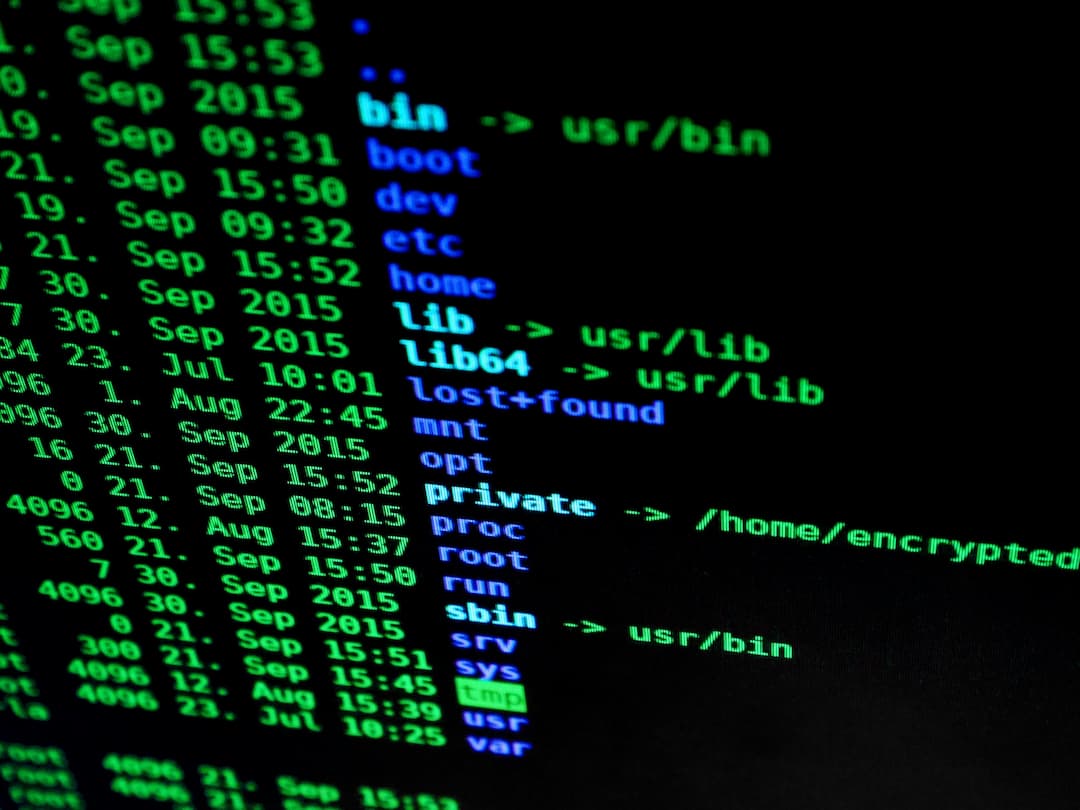
In the ever-evolving world of technology, privacy concerns are more prominent than ever. Imagine a world where your data never leaves your device, yet still contributes to the greater good. Welcome to the fascinating realm of federated learning! This cutting-edge approach to machine learning is redefining how we think about privacy and data security. Let’s dive into how federated learning ensures that your private information stays just that—private.
The Magic of Federated Learning
Federated learning flips the traditional model on its head. Instead of sending your data to a central server for processing, the machine learning model comes to you. Your device trains the model using your data locally, and only the updates (not your data) are sent back. This innovative method keeps your information safe and sound on your device.
The Secret Sauce: Key Privacy Mechanisms
1. Data Stays Home
With federated learning, your raw data never leaves your device. Whether it's your browsing history, health data, or personal messages, everything stays local. The model learns from your data on your device, and only the training results (model parameters) are sent back to the server.
2. Secure Aggregation
Even though your device sends updates to a central server, these updates are securely aggregated. Using advanced cryptographic protocols, the server can combine updates from thousands of devices without peeking into any single one. It's like mixing a giant batch of cookies without knowing the exact ingredients from each baker.
3. Differential Privacy
To add another layer of security, differential privacy sprinkles a bit of noise into the mix. This means that even if someone intercepts the model updates, they can’t decipher any specific user’s data. It's like adding static to a radio signal—still useful, but impossible to pick out individual voices.
4. Federated Averaging
When updates from your device and others are sent to the server, they’re averaged together. This averaging process ensures that individual contributions are blurred, making it even harder to trace updates back to a single user. Think of it as blending colors to get a unique shade—no single color stands out.
5. Encryption All the Way
Model updates are encrypted before they leave your device, ensuring they’re protected during transit. End-to-end encryption means that only the intended recipient—the central server—can read these updates, keeping prying eyes at bay.
Why Federated Learning is a Game-Changer
1. Data Breaches? Not Here
By keeping your data on your device, federated learning drastically reduces the risk of massive data breaches. Even if the central server gets hacked, there’s no raw data to steal.
2. Complying with Privacy Laws
Federated learning aligns with data protection regulations like GDPR by minimizing data transfer and storage. It’s a win-win: developers get to improve their models, and your data stays private.
3. Building Trust
People are more likely to use services they trust. By prioritizing privacy, federated learning helps build that trust, encouraging more users to engage with intelligent, data-driven applications.
Federated learning is paving the way for a new era of privacy-conscious technology, ensuring that your data remains yours while still contributing to advancements in machine learning. By embracing these innovative privacy mechanisms, federated learning not only protects your information but also fosters a more secure and trustworthy digital environment.